Why have SQL and NOSQL technologies failed to unlock the full potential of Analytics data?
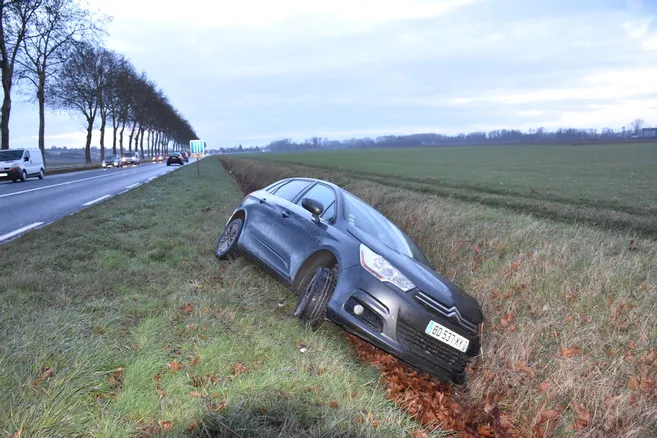
Why have SQL and NOSQL technologies failed to unlock the full potential of Analytics data?
Historically, control data originated in the Data Warehouse implemented in SQL-type databases in the 1980s.
Since SQL databases are fragile to change (adding SQL columns, adding SQL relationships, SQL relational constraints), increasing the scope of control data is expensive. This risk has caused a slowdown in the implementation of new data-driven indicators. The value provided by these always being challenged against the risk of implementation.
These limitations did cause Analytics data platforms to become frozen in time like a “statue of salt”. The potential of the data was limited without really being able to envisage a way out from this trap.
The new NOSQL technology emerged in 2005 to “explode the statue of salt”. This technology makes a reset on SQL constraints, it carries the promise of an expansion of business value without technical limitations.
NOSQL technology has favored the feeding of data in a massive and anarchic manner.
As business concepts and their links could no longer be modeled, the business value of these large data wells became elusive.
Edge effects quickly appeared. Several indicators manipulating the same business concepts could produce diverging values due to the developers' reinterpretation of the feeded data. Several indicators manipulating the same business concepts could use different terminology giving the illusion of vague concepts, difficult to interpret and above all difficult to correlate with other indicators. Another point, the implementation of a new indicator costs the total price each time because there is no recycling of all or part of the data already present on the platform.
The Cloud-ification of these platforms has often brought an end for massive feeding practices.
During the 2010s, the new Datavault technology appeared (even if the concepts had already been in place for more than ten years). This technology promises the representation of business concepts with their links while allowing unlimited expansion. In short, all the advantages of SQL and NOSQL technologies without any of their disadvantages.
Datavault technology is based on physical SQL modeling while seperating tables and columns. The result is a complex SQL schema that is expensive to implement.
Some solutions on the market offer automation of these aspects of physical modeling.
The virtues of automated Datavault are therefore the following:
• Business concepts and their links clearly materialized in a centralized manner (Datavault model), improved quality
• As the feed flows are dumped into the Datavault model, the values of the different indicators are consistent with each other, improved quality
• The creation of a new indicator takes advantage of the data already modeled and fed into the Datavault model, reduced time to product
• As the configuration of all these elements is automated (drag/drop with the mouse and very low code), the developers's team can be reduced down to 80%, reducing the budget
In conclusion, the new Datavault technology will succeed where SQL and NOSQL failed.
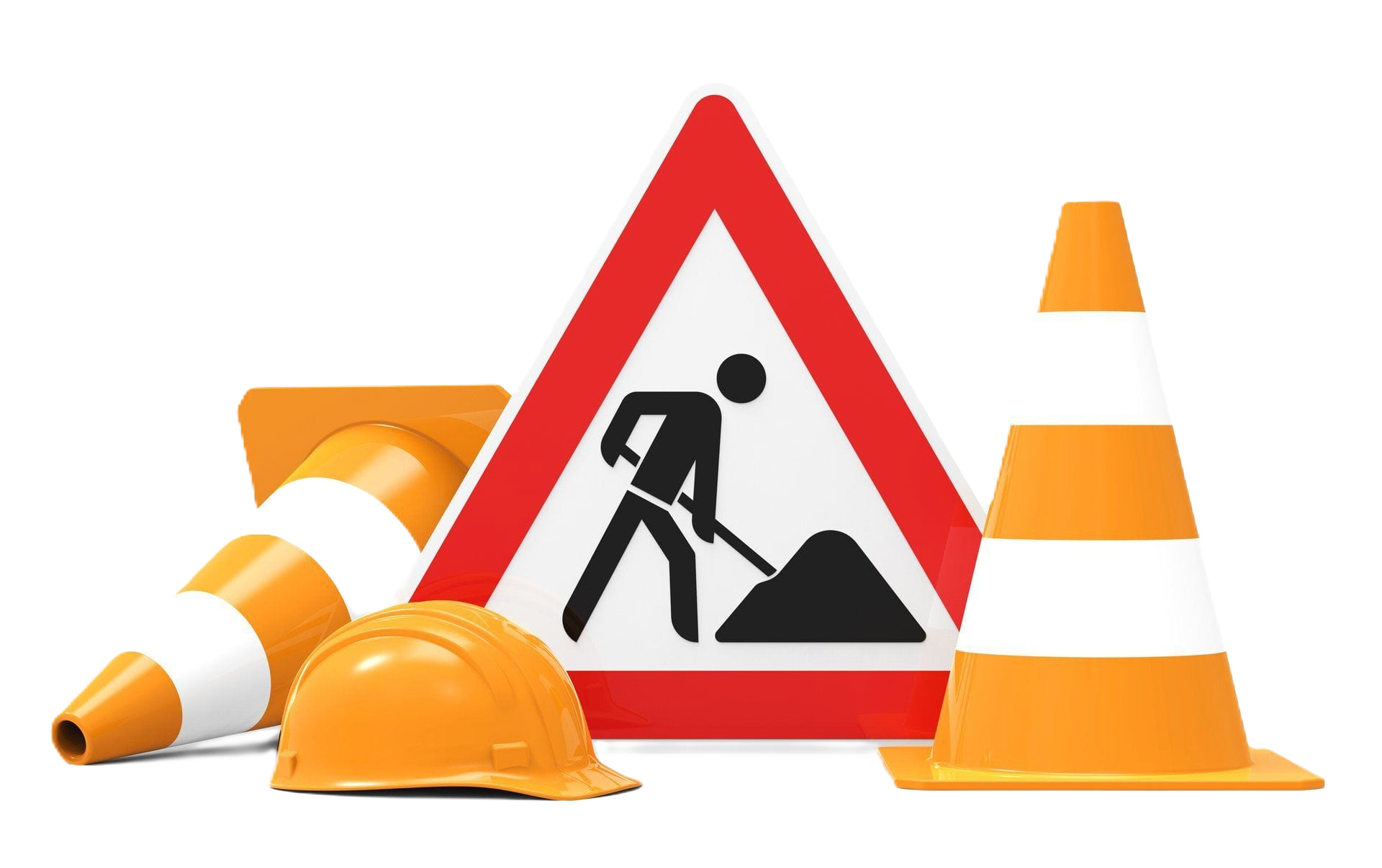
Translation in English under works
About the author
Page LinkedIn
José est expert Data et plus particulièrement Modélisation depuis une dizaine d'années. Auparavant, il a été architecte logiciel, API, SOAP ou encore Grille de Calculs. Il se passionne à batir une chaine de valeur industrialisé autour du Modèle de Données d'Entreprise.